The Independent's journalism is supported by our readers. When you purchase through links on our site, we may earn commission.
Surprise storms and chaos theory: How AI is about to cause a ‘weather revolution’
A model pioneered by the person who came up with the concept of the butterfly effect is still being used in forecasting systems, writes Anthony Cuthbertson. A new method uses similar tech to ChatGPT – and could be about to change weather prediction for ever
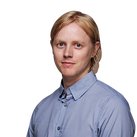
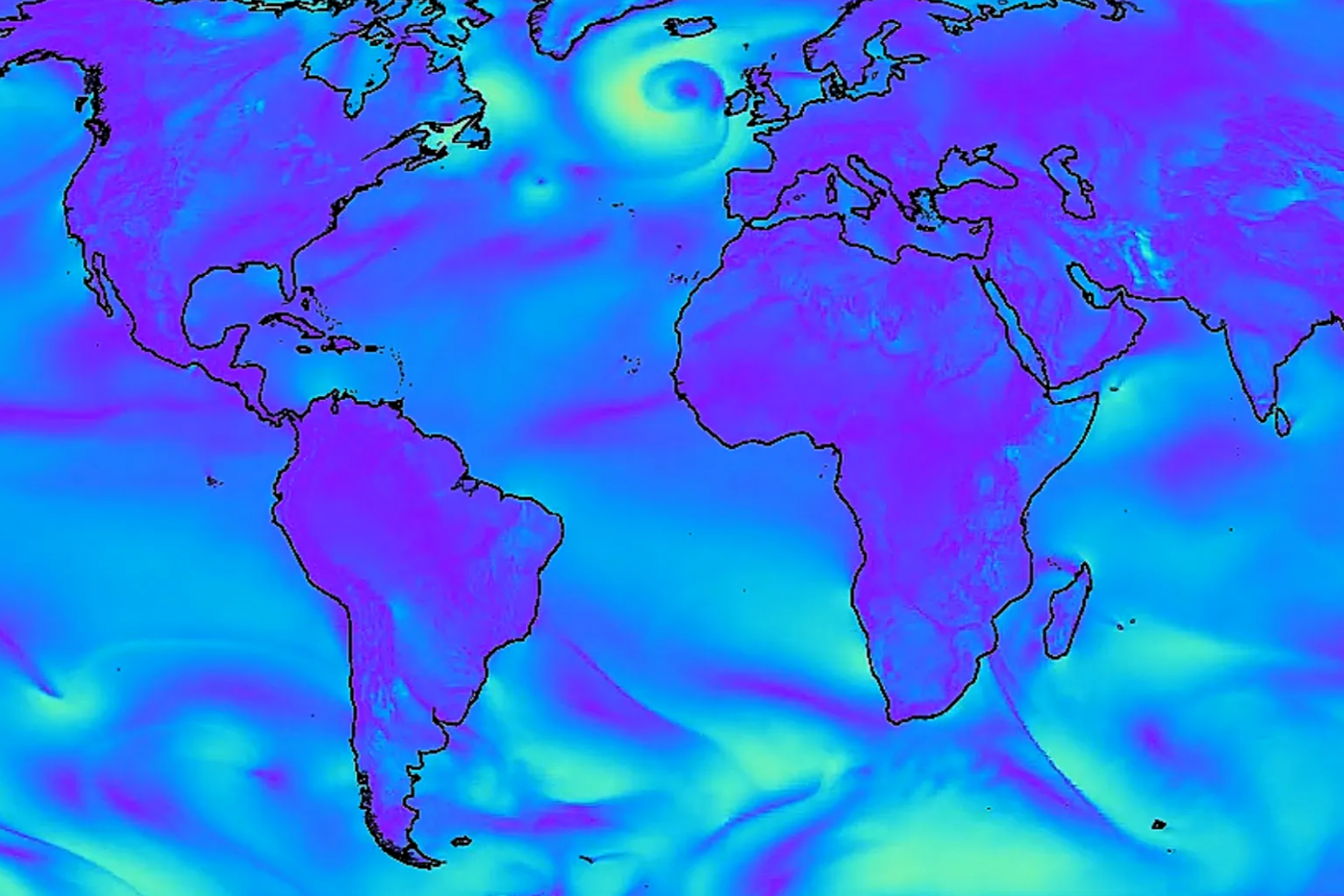
At 2.30am, Michael Fish was awoken by a tree blowing over in his back garden. It was 16 October 1987, and just 13 hours earlier, the weatherman had delivered what is now remembered as the UK’s most infamous forecast. “A woman rang the BBC and said she heard that there was a hurricane on the way,” he’d said during the previous lunchtime’s broadcast. “Well, if you’re watching, don’t worry, there isn’t.”
The hurricane-force winds that ensued ripped out not only Fish’s tree, but 15 million others across the south of England, destroyed billions of pounds’ worth of property, and killed 18 people. The next day, the front page of the Daily Mail featured the headline: “Why weren’t we warned?”
It was a reminder that not only is weather forecasting hard, it can be deadly when wrong. There have been significant improvements in the years since – most notably with the amount of computational power available to carry out weather simulations – yet the underlying prediction models remain the same.
This could be about to change in a big way. A rapid series of breakthroughs have uncovered brand new systems that can deliver unprecedented forecasting accuracy with just a fraction of the computing resources. And all in record time.
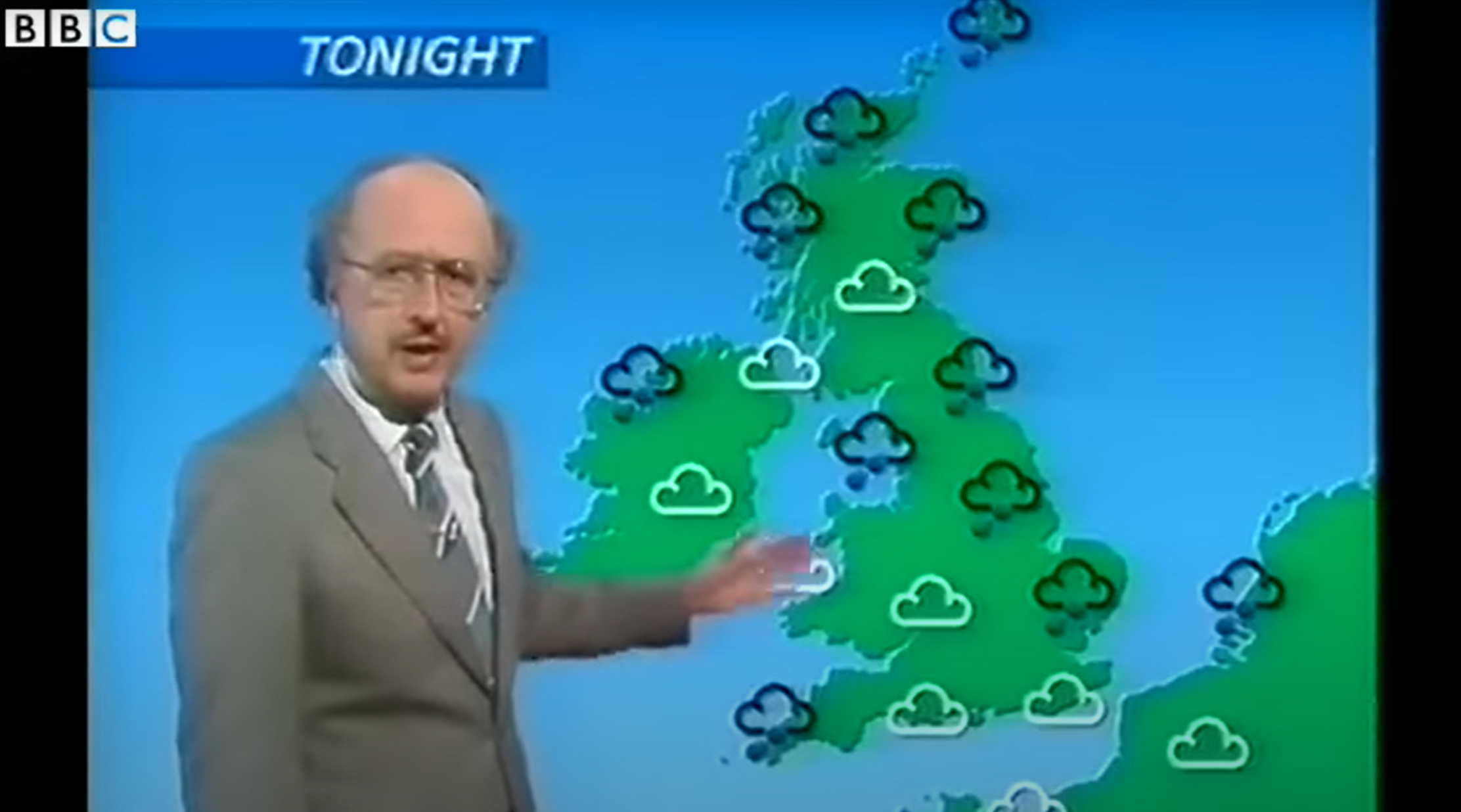
It began in 2020, when a scientific paper laid out a new method that was radically different from numerical weather prediction (NWP), which meteorologists have been using for decades to produce forecasts. NWP takes data from weather stations and satellites, and then uses complex equations involving things like fluid dynamics and wind turbulence to simulate how weather patterns might progress over time.
The process of mathematically replicating real-world physics is slow and expensive, requiring vast amounts of computing power to predict the weather, as well as experts to decipher the data – and it can still turn out to be wrong. (One of the pioneers of NWP, Edward Norton Lorenz, was also the founder of chaos theory. He famously expressed how tricky this deterministic, physics-based approach to weather was, in a paper he presented in 1972 titled “Does the flap of a butterfly’s wings in Brazil set off a tornado in Texas?”)
Frustrated with this approach, researchers at the University of Washington in the US came up with a way to generate near-instant forecasts using artificial intelligence. They found that AI statistical models trained on decades of forecasts – similar to how ChatGPT is trained on vast amounts of text data – were able to predict how the weather would develop using real-time weather conditions.
“Machine learning is essentially doing a glorified version of pattern recognition,” says Jonathan Weyn, who led the research. “It sees a typical pattern, recognises how it usually evolves, and decides what to do based on the examples it has seen in the past 40 years of data.”
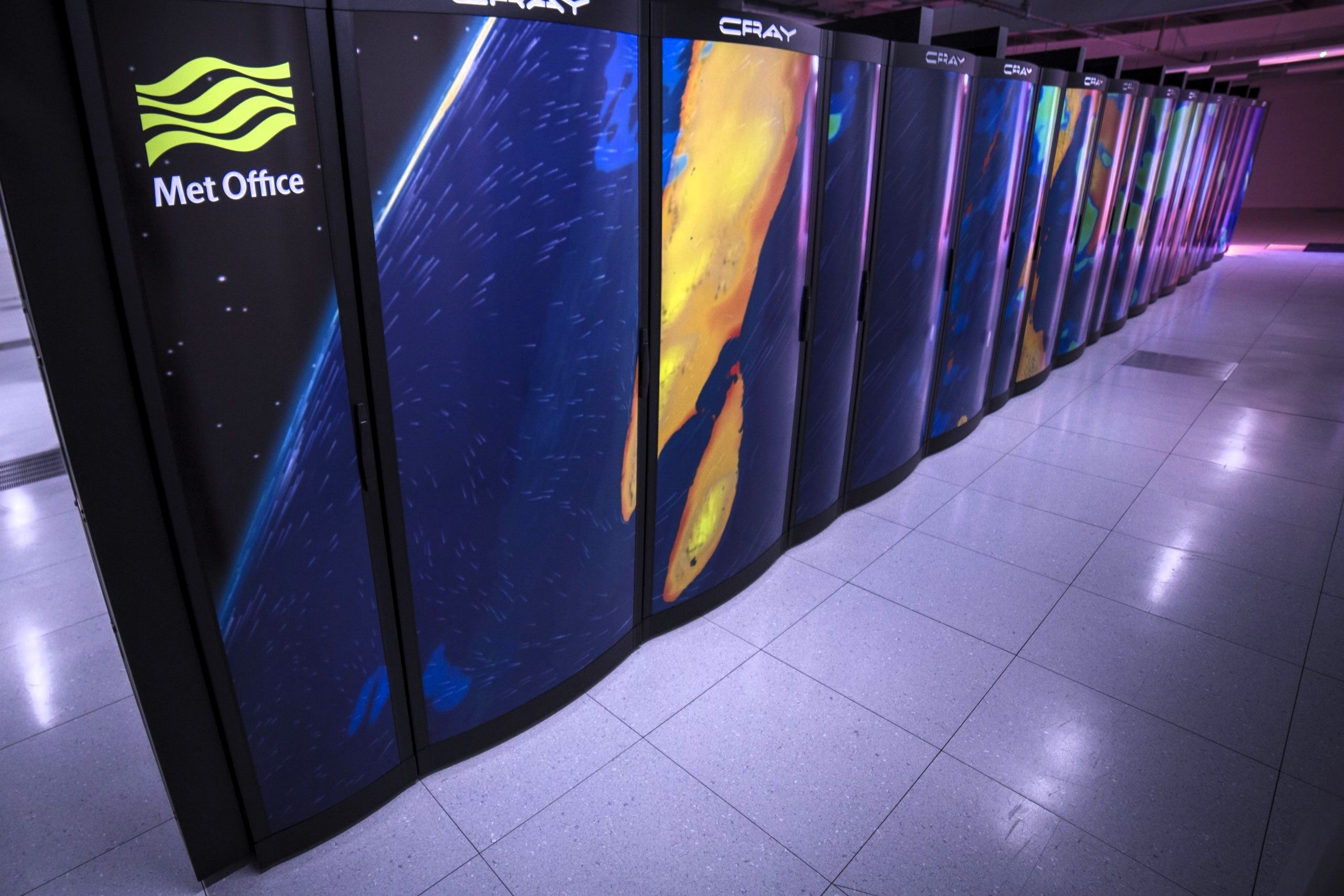
The AI model Weyn’s team built in 2020 was not as accurate as the best traditional forecasting models, but remarkably, it used 7,000 times less computing power to create its forecasts. It formed the basis of more advanced AI models that Weyn continued to develop at Microsoft, as well as those built by other tech giants like Google DeepMind.
By 2023, the models were proving more accurate over short periods than traditional forecasting methods. That year, DeepMind’s GraphCast system produced a 10-day forecast in under a minute using just one machine – a process that would otherwise take hours on a supercomputer. A paper published in the journal Science revealed that it was more accurate than the best NWP systems in 90 per cent of tests.
Recent leaps in AI development have been reflected in the advances made in forecasting. In March, Cambridge researchers unveiled a new model called Aardvark, which can outperform the US National Oceanic and Atmospheric Administration’s (NOAA) global forecast system while using just 10 per cent of the input data.
Meteorologists described it as a “revolution in forecasting” that would allow anyone to create a personalised weather report from their smartphone.
“Aardvark’s breakthrough is not just about speed, it’s about access,” says Dr Scott Hosking, director of science and innovation at the Alan Turing Institute in London. “By shifting weather prediction from supercomputers to desktop computers, we can democratise forecasting, making these powerful technologies available to developing nations and data-sparse regions around the world.”
Potential applications range from optimising the output of offshore wind farms to providing precise rainfall projections for farmers in drought-stricken areas.
Perhaps most importantly, this new AI approach is proving unparalleled at predicting chaos. As early as 2023, DeepMind’s GraphCast was able to outline the path of Hurricane Lee nine days before it arrived, despite not even being trained on data from extreme weather events. Traditional forecasts took an extra three days to detect it.
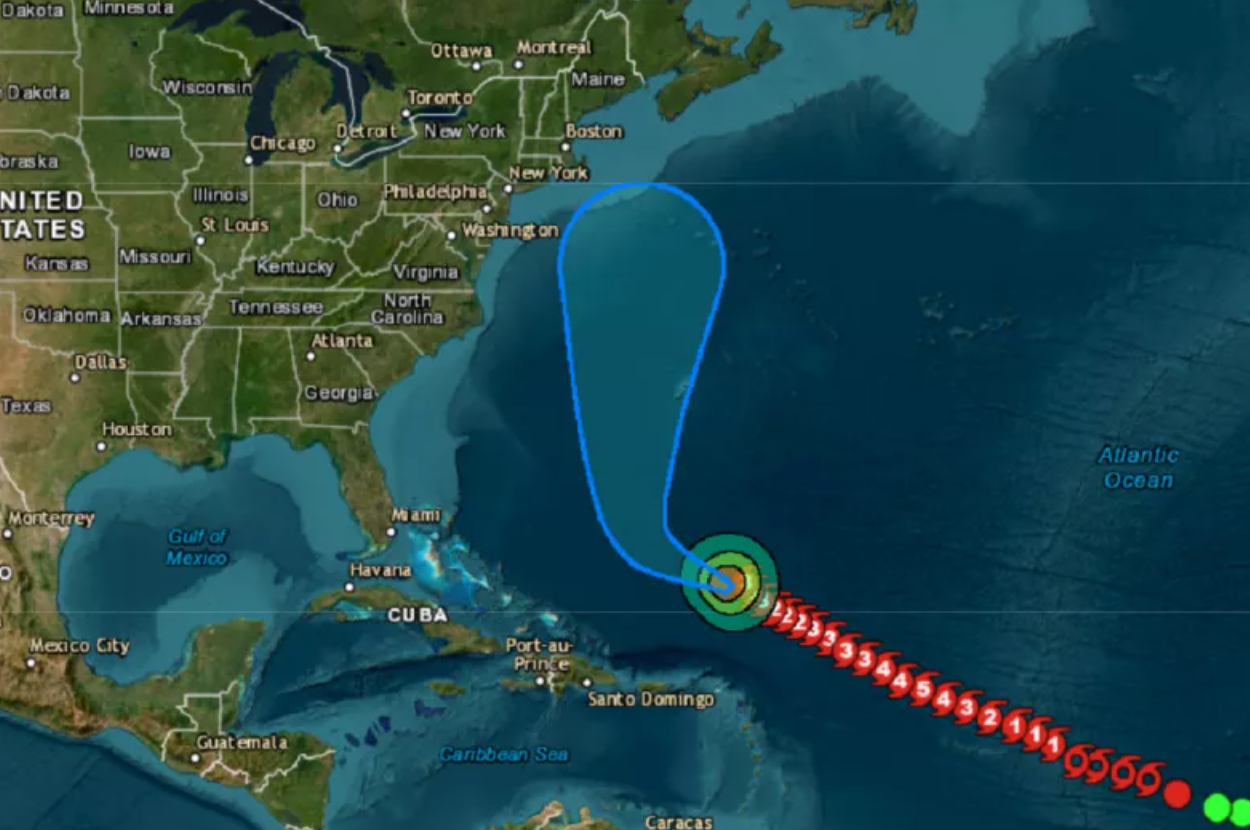
AI models are also proving useful in predicting wildfires, with the European Centre for Medium-Range Weather Forecasts (ECMWF) unveiling an algorithm this week that analyses fire patterns, potential fuel sources and human activity to predict which areas might be at risk. A test run on data from the January 2025 blaze in southern California proved it to be better than current methods at identifying where fires would ignite.
There are still some obstacles to adopting the technology. Researchers in the US revealed this week that AI is sometimes unable to tell the difference between rain and snow when using only surface weather data.
“AI models still struggle between 0 degrees Celsius and 4C because rain and snow share nearly identical meteorological conditions at these temperatures,” says Wei Zhang, a climate scientist at Utah State University who contributed to the research. “We need more diverse data and physical variables to improve predictions.”
There may also be a reluctance to abandon traditional methods. The Met Office, for example, invested £1.2bn in a state-of-the-art supercomputer for weather and climate forecasting in February 2020 – just six months before the first big AI breakthrough – meaning that the new approach will likely complement existing systems until they become completely redundant.
NOAA in the US and the ECMWF have already begun integrating AI models into their existing forecasting kits, and even the Met Office has teamed up with the Alan Turing Institute and hired a chief AI officer to better understand how artificial intelligence can be used to improve weather prediction – and avoid a repeat of Fish’s fateful forecast ahead of the great storm of 1987.
At an event in November announcing a new machine-learning model developed in partnership with the Turing Institute, the Met Office’s new chief AI officer, Kirstine Dale, said AI would prove crucial for coping with the ever-increasing extreme weather events caused by climate change.
“There are some major environmental challenges facing us, and we’re increasingly aware of our vulnerability to extremes in the weather,” she said. “We’re in the midst of an AI revolution, and it’s happening at just the right time.”
Join our commenting forum
Join thought-provoking conversations, follow other Independent readers and see their replies
Comments